《数据仓库与数据挖掘》第8章.pptx
《《数据仓库与数据挖掘》第8章.pptx》由会员分享,可在线阅读,更多相关《《数据仓库与数据挖掘》第8章.pptx(148页珍藏版)》请在冰点文库上搜索。
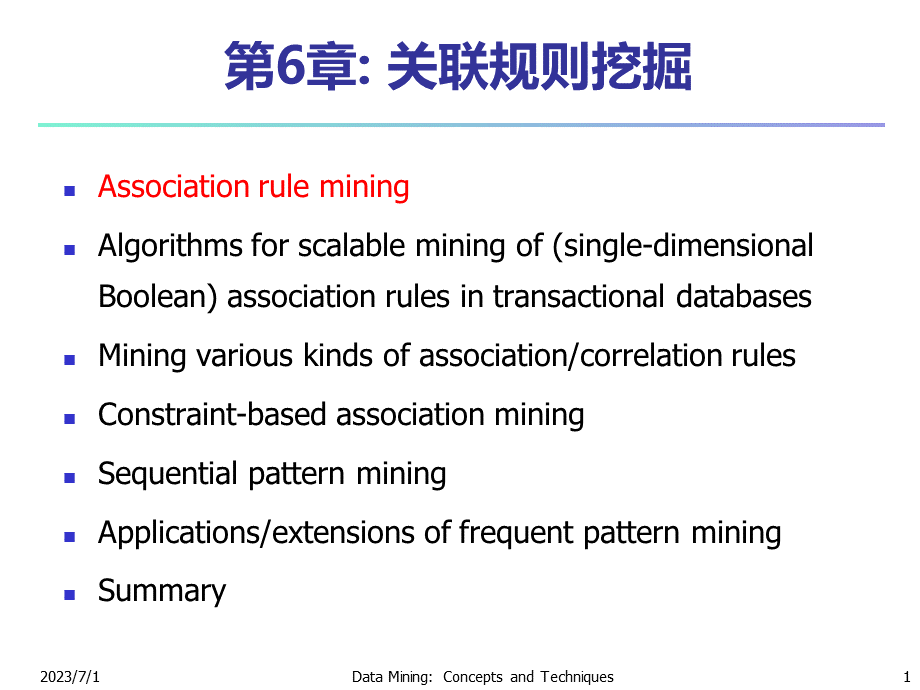
第6章:
关联规则挖掘,AssociationruleminingAlgorithmsforscalableminingof(single-dimensionalBoolean)associationrulesintransactionaldatabasesMiningvariouskindsofassociation/correlationrulesConstraint-basedassociationminingSequentialpatternminingApplications/extensionsoffrequentpatternminingSummary,2023/7/1,1,DataMining:
ConceptsandTechniques,WhatIsAssociationMining?
Associationrulemining:
Findingfrequentpatterns,associations,correlations,orcausalstructuresamongsetsofitemsorobjectsintransactiondatabases,relationaldatabases,andotherinformationrepositories.Frequentpattern:
pattern(setofitems,sequence,etc.)thatoccursfrequentlyinadatabaseAIS93Motivation:
findingregularitiesindataWhatproductswereoftenpurchasedtogether?
Beeranddiapers?
!
WhatarethesubsequentpurchasesafterbuyingaPC?
WhatkindsofDNAaresensitivetothisnewdrug?
Canweautomaticallyclassifywebdocuments?
2023/7/1,2,DataMining:
ConceptsandTechniques,关联规则挖掘的基本概念,购物篮分析引发关联规则挖掘的例子问题:
“什么商品组或集合顾客多半会在一次购物中同时购买?
”购物篮分析:
设全域为商店出售的商品的集合(即项目全集),一次购物购买(即事务)的商品为项目全集的子集,若每种商品用一个布尔变量表示该商品的有无,则每个购物篮可用一个布尔向量表示。
通过对布尔向量的分析,得到反映商品频繁关联或同时购买的购买模式。
这些模式可用关联规则描述。
例购买计算机与购买财务管理软件的关联规则可表示为:
computerfinancial_management_softwarsupport=2%,confidence=60%support为支持度,confidence为置信度。
该规则表示:
在所分析的全部事务中,有2的事务同时购买计算机和财务管理软件;在购买计算机的顾客中60也购买财务管理软件。
2023/7/1,3,DataMining:
ConceptsandTechniques,WhyIsFrequentPatternorAssoiciationMininganEssentialTaskinDataMining?
FoundationformanyessentialdataminingtasksAssociation,correlation,causalitySequentialpatterns,temporalorcyclicassociation,partialperiodicity,spatialandmultimediaassociationAssociativeclassification,clusteranalysis,icebergcube,fascicles(semanticdatacompression)BroadapplicationsBasketdataanalysis,cross-marketing,catalogdesign,salecampaignanalysisWeblog(clickstream)analysis,DNAsequenceanalysis,etc.,2023/7/1,4,DataMining:
ConceptsandTechniques,关联规则,关联(Associations)分析的目的是为了挖掘隐藏在数据间的相互关系,即对于给定的一组项目和一个记录集,通过对记录集的分析,得出项目集中的项目之间的相关性。
项目之间的相关性用关联规则来描述,关联规则反映了一组数据项之间的密切程度或关系。
定义81令I=i1,i2,,in是项目集,D是全体事务的集合。
事务T是I上的一个子集,集合TI,每个事务用唯一的标志TID来标识。
关联规则是形如XY的蕴含式,其中XI,YI且XY=,X称为规则的条件,Y称为规则的结果。
2023/7/1,5,DataMining:
ConceptsandTechniques,置信度和支持度,定义82关联规则XY对事物集D的支持度(support,)定义为D中包含有事务X和Y的百分比。
关联规则XY对事务集合D的置信度(confidence)定义为D中包含有X的事务数与同时包含Y的百分比。
即:
support(XY)(包含X和Y的事务数/事务总数)100confidence(XY)包含X和Y的事务数/包含X的事务数)100定义83置信度和支持度均大于给定阈值(即最小置信度阈值和最小支持度阈值)。
即:
support(XY)min_supconfidence(XY)min_conf的关联规则称为强规则;否则称为弱规则。
2023/7/1,6,DataMining:
ConceptsandTechniques,关联规则挖掘,数据挖掘主要就是对强规则的挖掘。
通过设置最小支持度和最小置信度可以了解某些数据之间的关联程度。
关联规则挖掘:
给定一组Item和记录集合,挖掘出Item间的相关性,使其置信度和支持度分别大于用户给定的最小置信度和、最小支持度。
2023/7/1,7,DataMining:
ConceptsandTechniques,关联规则挖掘,数据挖掘主要就是对强规则的挖掘。
通过设置最小支持度和最小置信度可以了解某些数据之间的关联程度。
强规则XY对应的项集(XY)必定是频繁集。
因此,可以把关联规则挖掘划分为以下两个子问题:
根据最小支持度找出事务集D中的所有频繁项集。
核心根据频繁项集和最小置信度产生关联规则。
较易关联规则挖掘:
给定一组Item和记录集合,挖掘出Item间的相关性,使其置信度和支持度分别大于用户给定的最小置信度和、最小支持度。
2023/7/1,8,DataMining:
ConceptsandTechniques,关联规则挖掘的分类基于变量的类别,基于规则中处理的变量的类别,关联规则可以分为布尔型和数值型:
布尔型关联规则:
如果规则考虑的关联是项“在”或“不在”,则关联规则是布尔型的。
例如,由购物篮分析得出的关联规则。
量化型关联规则:
如果描述的是量化的项或属性之间的关联,则该规则是量化型的关联规则。
例如,以下是量化型关联规则的一个例子(其中X为表示顾客的变量,量化属性age和income已经离散化):
age(X,“3039”)income(“42K48K”)buys(X,“high_resolution_TV”)量化型关联规则中也可以包含多种变量。
例如:
性别=“女”=职业=“秘书”,是布尔型关联规则;性别=“女”=avg(月收入)=2300,涉及的收入是数值类型,所以是一个量化型关联规则。
2023/7/1,9,DataMining:
ConceptsandTechniques,关联规则挖掘的分类基于抽象层次,基于规则中数据的抽象层次,可以分为单层关联规则和多层关联规则:
单层的关联规则:
所有的变量都不涉及不同抽象层次的项或属性。
例如:
buys(X,“computer”)buys(X,“printer”)顾客X购买的商品不涉及不同抽象层次(“computer”和“printer”在同一个抽象层),因此是单层关联规则。
多层的关联规则:
变量涉及不同抽象层次的项或属性。
例如:
age(X,“3039”)buys(X,“laptopcomputer”)age(X,“3039”)buys(X,“computer”)顾客X购买的商品涉及不同抽象层次(“computer”在比“laptopcomputer”高的抽象层),因此是多层关联规则。
2023/7/1,10,DataMining:
ConceptsandTechniques,关联规则挖掘的分类基于数据的维数,基于规则中涉及到的数据的维数,关联规则可以分为单维的和多维的单维关联规则:
处理单个维中属性间的关系,即在单维的关联规则中,只涉及到数据的一个维。
例如:
用户购买的物品:
“咖啡=砂糖”,这条规则只涉及到用户的购买的物品。
多维关联规则:
处理多个维中属性之间的关系,即在多维的关联规则中,要处理的数据将会涉及多个维。
例如:
性别=“女”=职业=“秘书”,这条规则就涉及到两个维中字段的信息,是两个维上的一条关联规则,2023/7/1,11,DataMining:
ConceptsandTechniques,关联规则挖掘的过程,定义84在关联规则挖掘算法中,把项目的集合称为项集(itemset),包含有k个项目的项集称为k-项集。
包含项集的事务数称为项集的出现频率,简称为项集的频率或支持度计数。
如果项集的出现频率大于或等于最小支持度s与D中事务总数的乘积,则称该项集满足最小支持度s。
如果项集满足最小支持度,则称该项集为频繁项集(frequentitemset)。
关联规则的挖掘主要被分解为下面两步:
第1步:
找出所有的频繁项集,即找出支持度大于或等于给定的最小支持度阈值的所有项集。
可以从1到k递归查找k-频繁项集。
第2步:
由频繁项集产生强关联规则,即找出满足最小支持度和最小置信度的关联规则。
对给定的L,如果其非空子集AL,sup(L)为L的支持度,sup(A)为A的支持度,则产生形式为AL-A的规则。
2023/7/1,12,DataMining:
ConceptsandTechniques,BasicConcepts:
FrequentPatternsandAssociationRules,ItemsetX=x1,xkFindalltherulesXYwithminconfidenceandsupportsupport,s,probabilitythatatransactioncontainsXYconfidence,c,conditionalprobabilitythatatransactionhavingXalsocontainsY.,Letmin_support=50%,min_conf=50%:
AC(50%,66.7%)CA(50%,100%),2023/7/1,13,DataMining:
ConceptsandTechniques,MiningAssociationRulesanExample,ForruleAC:
support=support(AC)=50%confidence=support(AC)/support(A)=66.6%,Min.support50%Min.confidence50%,2023/7/1,14,DataMining:
ConceptsandTechniques,Chapter6:
MiningAssociationRulesinLargeDatabases,AssociationruleminingAlgorithmsforscalableminingof(single-dimensionalBoolean)associationrulesintransactionaldatabasesMiningvariouskindsofassociation/correlationrulesConstraint-basedassociationminingSequentialpatternminingApplications/extensionsoffrequentpatternminingSummary,2023/7/1,15,DataMining:
ConceptsandTechniques,Apriori:
ACandidateGeneration-and-testApproach,Anysubsetofafrequentitemsetmustbefrequentifbeer,diaper,nutsisfrequent,soisbeer,diaperEverytransactionhavingbeer,diaper,nutsalsocontainsbeer,diaperAprioripruningprinciple:
Ifthereisanyitemsetwhichisinfrequent,itssupersetshouldnotbegenerated/tested!
Method:
generatelength(k+1)candidateitemsetsfromlengthkfrequentitemsets,andtestthecandidatesagainstDBTheperformancestudiesshowitsefficiencyandscalabilityAgrawal&Srikant1994,Mannila,etal.1994,2023/7/1,16,DataMining:
ConceptsandTechniques,TheAprioriAlgorithmAnExample,DatabaseTDB,1stscan,C1,L1,L2,C2,C2,2ndscan,C3,L3,3rdscan,2023/7/1,17,DataMining:
ConceptsandTechniques,TheAprioriAlgorithm,Pseudo-code:
Ck:
CandidateitemsetofsizekLk:
frequentitemsetofsizekL1=frequentitems;for(k=1;Lk!
=;k+)dobeginCk+1=candidatesgeneratedfromLk;foreachtransactiontindatabasedoincrementthecountofallcandidatesinCk+1thatarecontainedintLk+1=candidatesinCk+1withmin_supportendreturnkLk;,2023/7/1,18,DataMining:
ConceptsandTechniques,ImportantDetailsofApriori,Howtogeneratecandidates?
Step1:
self-joiningLkStep2:
pruningHowtocountsupportsofcandidates?
ExampleofCandidate-generationL3=abc,abd,acd,ace,bcdSelf-joining:
L3*L3abcdfromabcandabdacdefromacdandacePruning:
acdeisremovedbecauseadeisnotinL3C4=abcd,2023/7/1,19,DataMining:
ConceptsandTechniques,HowtoGenerateCandidates?
SupposetheitemsinLk-1arelistedinanorderStep1:
self-joiningLk-1insertintoCkselectp.item1,p.item2,p.itemk-1,q.itemk-1fromLk-1p,Lk-1qwherep.item1=q.item1,p.itemk-2=q.itemk-2,p.itemk-1q.itemk-1Step2:
pruningforallitemsetscinCkdoforall(k-1)-subsetssofcdoif(sisnotinLk-1)thendeletecfromCk,2023/7/1,20,DataMining:
ConceptsandTechniques,HowtoCountSupportsofCandidates?
Whycountingsupportsofcandidatesaproblem?
ThetotalnumberofcandidatescanbeveryhugeOnetransactionmaycontainmanycandidatesMethod:
Candidateitemsetsarestoredinahash-treeLeafnodeofhash-treecontainsalistofitemsetsandcountsInteriornodecontainsahashtableSubsetfunction:
findsallthecandidatescontainedinatransaction,2023/7/1,21,DataMining:
ConceptsandTechniques,Example:
CountingSupportsofCandidates,Transaction:
12356,1+2356,12+356,13+56,2023/7/1,22,DataMining:
ConceptsandTechniques,由频繁项集而产生关联规则,一旦数据库D中的事务找出的频繁项集,由它们产生强关联规则是直截了当的。
置信度使用下式计算:
Confidence(AB)=support_count(AB)/support_count(A)其中:
support_count(AB)是包含AB的事务数,support_count(A)是包含A的事务数。
关联规则产生的步骤:
第1步:
对于每一个频繁项集I,产生I的所有非空子集。
第2步:
对于I的每一个非空子集s,如果support_count(I)/support_count(s)=min_conf则输出关联规则“s(I-s)”,其中min_conf为最小置信度阈值。
2023/7/1,23,DataMining:
ConceptsandTechniques,由频繁项集而产生关联规则,例假设数据包含频繁项集I=I1,I2,I5:
第1步:
对于频繁项集I=I1,I2,I5,产生I的所有非空子集:
I1,I2,I1,I5,I2,I5,I1,I2,I5第2步:
对于I的每一个非空子集s,输出关联规则“s(I-s)”I1I2I5confidence=2/4=50%I1I5I2confidence=2/2=100%I2I5I1confidence=2/2=100%I1I2I5confidence=2/6=33%I2I1I5confidence=2/7=29%I5I1I2confidence=2/7=100%如果最小置信度设定为70,则只有以下三个关联规则输出:
I1I5I2confidence=2/2=100%I2I5I1confidence=2/2=100%I5I1I2confidence=2/7=100%,2023/7/1,24,DataMining:
ConceptsandTechniques,例子,例以下表所示的事务集为例,其中Ci是候选集,Li是大数据项集。
假设最小支持度为40%,最小置信度为70%。
则数据项在候选集中至少要出现4次以上才能满足大数据项的条件,规则的可信度至少要大于70%才能形成关联规则。
Apriori关联规则挖掘过程如图所示。
2023/7/1,25,DataMining:
ConceptsandTechniques,ChallengesofFrequentPatternMining,ChallengesMultiplescansoftransactiondatabaseHugenumberofcandidatesTediousworkloadofsupportcountingforcandidatesImprovingApriori:
generalideasReducepassesoftransactiondatabasescansShrinknumberofcandidatesFacilitatesupportcountingofcandidates,2023/7/1,26,DataMining:
ConceptsandTechniques,DIC:
ReduceNumberofScans,ABCD,ABC,ABD,ACD,BCD,AB,AC,BC,AD,BD,CD,A,B,C,D,Itemsetlattice,OncebothAandDaredeterminedfrequent,thecountingofADbeginsOncealllength-2subsetsofBCDaredeterminedfrequent,thecountingofBCDbegins,Transactions,1-itemsets,2-itemsets,Apriori,1-itemsets,2-items,3-items,DIC,S.BrinR.Motwani,J.Ullman,andS.Tsur.Dynamicitemsetcountingandimplicationrulesformarketbasketdata.InSIGMOD97,2023/7/1,27,DataMining:
ConceptsandTechniques,Partition:
ScanDatabaseOnlyTwice,AnyitemsetthatispotentiallyfrequentinDBmustbefrequentinatleastoneofthepartitionsofDBScan1:
partitiondatabaseandfindlocalfrequentpatternsScan2:
consolidateglobalfrequentpatternsA.Savasere,E.Omiecinski,andS.Navathe.Anefficientalgorithmforminingassociationinlargedatabases.InVLDB95,2023/7/1,28,DataMining:
ConceptsandTechniques,Sa