scale space.docx
《scale space.docx》由会员分享,可在线阅读,更多相关《scale space.docx(11页珍藏版)》请在冰点文库上搜索。
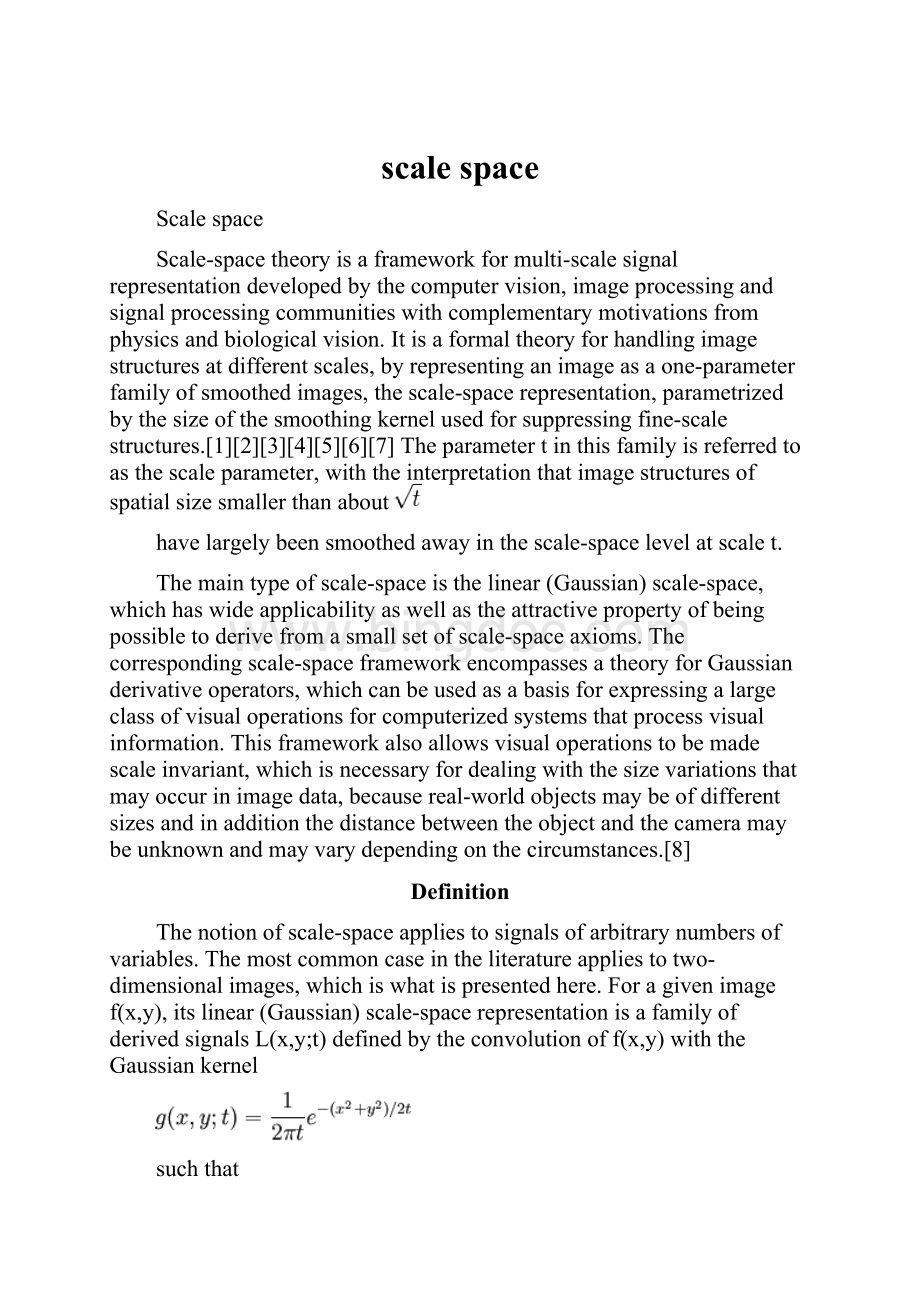
scalespace
Scalespace
Scale-spacetheoryisaframeworkformulti-scalesignalrepresentationdevelopedbythecomputervision,imageprocessingandsignalprocessingcommunitieswithcomplementarymotivationsfromphysicsandbiologicalvision.Itisaformaltheoryforhandlingimagestructuresatdifferentscales,byrepresentinganimageasaone-parameterfamilyofsmoothedimages,thescale-spacerepresentation,parametrizedbythesizeofthesmoothingkernelusedforsuppressingfine-scalestructures.[1][2][3][4][5][6][7]Theparametertinthisfamilyisreferredtoasthescaleparameter,withtheinterpretationthatimagestructuresofspatialsizesmallerthanabout
havelargelybeensmoothedawayinthescale-spacelevelatscalet.
Themaintypeofscale-spaceisthelinear(Gaussian)scale-space,whichhaswideapplicabilityaswellastheattractivepropertyofbeingpossibletoderivefromasmallsetofscale-spaceaxioms.Thecorrespondingscale-spaceframeworkencompassesatheoryforGaussianderivativeoperators,whichcanbeusedasabasisforexpressingalargeclassofvisualoperationsforcomputerizedsystemsthatprocessvisualinformation.Thisframeworkalsoallowsvisualoperationstobemadescaleinvariant,whichisnecessaryfordealingwiththesizevariationsthatmayoccurinimagedata,becausereal-worldobjectsmaybeofdifferentsizesandinadditionthedistancebetweentheobjectandthecameramaybeunknownandmayvarydependingonthecircumstances.[8]
Definition
Thenotionofscale-spaceappliestosignalsofarbitrarynumbersofvariables.Themostcommoncaseintheliteratureappliestotwo-dimensionalimages,whichiswhatispresentedhere.Foragivenimagef(x,y),itslinear(Gaussian)scale-spacerepresentationisafamilyofderivedsignalsL(x,y;t)definedbytheconvolutionoff(x,y)withtheGaussiankernel
suchthat
wherethesemicolonintheargumentofLimpliesthattheconvolutionisperformedonlyoverthevariablesx,y,whilethescaleparametertafterthesemicolonjustindicateswhichscalelevelisbeingdefined.ThisdefinitionofLworksforacontinuumofscales
buttypicallyonlyafinitediscretesetoflevelsinthescale-spacerepresentationwouldbeactuallyconsidered.
tisthevarianceoftheGaussianfilterandasalimitfort=0thefiltergbecomesanimpulsefunctionsuchthatL(x,y;0)=f(x,y),thatis,thescale-spacerepresentationatscalelevelt=0istheimagefitself.Astincreases,Listheresultofsmoothingfwithalargerandlargerfilter,therebyremovingmoreandmoreofthedetailswhichtheimagecontains.Sincethestandarddeviationofthefilteris
detailswhicharesignificantlysmallerthanthisvaluearetoalargeextentremovedfromtheimageatscaleparametert,seethefollowingfigureand[9]forgraphicalillustrations.
∙
Scale-spacerepresentationL(x,y;t)atscalet=0,correspondingtotheoriginalimagef
∙
Scale-spacerepresentationL(x,y;t)atscalet=1
∙
Scale-spacerepresentationL(x,y;t)atscalet=4
∙
Scale-spacerepresentationL(x,y;t)atscalet=16
∙
Scale-spacerepresentationL(x,y;t)atscalet=64
∙
Scale-spacerepresentationL(x,y;t)atscalet=256
[edit]WhyaGaussianfilter?
Whenfacedwiththetaskofgeneratingamulti-scalerepresentationonemayask:
Couldanyfiltergoflow-passtypeandwithaparametertwhichdeterminesitswidthbeusedtogenerateascale-space?
Theanswerisno,asitisofcrucialimportancethatthesmoothingfilterdoesnotintroducenewspuriousstructuresatcoarsescalesthatdonotcorrespondtosimplificationsofcorrespondingstructuresatfinerscales.Inthescale-spaceliterature,anumberofdifferentwayshavebeenexpressedtoformulatethiscriterioninprecisemathematicalterms.
TheconclusionfromseveraldifferentaxiomaticderivationsthathavebeenpresentedisthattheGaussianscale-spaceconstitutesthecanonicalwaytogeneratealinearscale-space,basedontheessentialrequirementthatnewstructuresmustnotbecreatedwhengoingfromafinescaletoanycoarserscale.[2][3][5][8][10][11][12][13][14][15]Conditions,referredtoasscale-spaceaxioms,thathavebeenusedforderivingtheuniquenessoftheGaussiankernelincludelinearity,shiftinvariance,semi-groupstructure,non-enhancementoflocalextrema,scaleinvarianceandrotationalinvariance.
Equivalently,thescale-spacefamilycanbedefinedasthesolutionofthediffusionequation(forexampleintermsoftheheatequation),
withinitialconditionL(x,y;0)=f(x,y).Thisformulationofthescale-spacerepresentationLmeansthatitispossibletointerprettheintensityvaluesoftheimagefasa"temperaturedistribution"intheimageplaneandthattheprocesswhichgeneratesthescale-spacerepresentationasafunctionoftcorrespondstoheatdiffusionintheimageplaneovertimet(assumingthethermalconductivityofthematerialequaltothearbitrarilychosenconstant½).Althoughthisconnectionmayappearsuperficialforareadernotfamiliarwithdifferentialequations,itisindeedthecasethatthemainscale-spaceformulationintermsofnon-enhancementoflocalextremaisexpressedintermsofasignconditiononpartialderivativesinthe2+1-Dvolumegeneratedbythescale-space,thuswithintheframeworkofpartialdifferentialequations.Furthermore,adetailedanalysisofthediscretecaseshowsthatthediffusionequationprovidesaunifyinglinkbetweencontinuousanddiscretescale-spaces,whichalsogeneralizestonon-linearscale-spaces,forexample,usinganisotropicdiffusion.Hence,onemaysaythattheprimarywaytogenerateascale-spaceisbythediffusionequation,andthattheGaussiankernelarisesastheGreen'sfunctionofthisspecificpartialdifferentialequation.
Motivations
Themotivationforgeneratingascale-spacerepresentationofagivendatasetoriginatesfromthebasicobservationthatreal-worldobjectsarecomposedofdifferentstructuresatdifferentscales.Thisimpliesthatreal-worldobjects,incontrasttoidealizedmathematicalentitiessuchaspointsorlines,mayappearindifferentwaysdependingonthescaleofobservation.Forexample,theconceptofa"tree"isappropriateatthescaleofmeters,whileconceptssuchasleavesandmoleculesaremoreappropriateatfinerscales.Foracomputervisionsystemanalysinganunknownscene,thereisnowaytoknowaprioriwhatscalesareappropriatefordescribingtheinterestingstructuresintheimagedata.Hence,theonlyreasonableapproachistoconsiderdescriptionsatmultiplescalesinordertobeabletocapturetheunknownscalevariationsthatmayoccur.Takentothelimit,ascale-spacerepresentationconsidersrepresentationsatallscales.[8]
Anothermotivationtothescale-spaceconceptoriginatesfromtheprocessofperformingaphysicalmeasurementonreal-worlddata.Inordertoextractanyinformationfromameasurementprocess,onehastoapplyoperatorsofnon-infinitesimalsizetothedata.Inmanybranchesofcomputerscienceandappliedmathematics,thesizeofthemeasurementoperatorisdisregardedinthetheoreticalmodellingofaproblem.Thescale-spacetheoryontheotherhandexplicitlyincorporatestheneedforanon-infinitesimalsizeoftheimageoperatorsasanintegralpartofanymeasurementaswellasanyotheroperationthatdependsonareal-worldmeasurement.[4]
Thereisacloselinkbetweenscale-spacetheoryandbiologicalvision.Manyscale-spaceoperationsshowahighdegreeofsimilaritywithreceptivefieldprofilesrecordedfromthemammalianretinaandthefirststagesinthevisualcortex.Intheserespects,thescale-spaceframeworkcanbeseenasatheoreticallywell-foundedparadigmforearlyvision,whichinadditionhasbeenthoroughlytestedbyalgorithmsandexperiments.[3][8]
[edit]Gaussianderivativesandthenotionofavisualfront-end
Atanyscaleinscale-space,wecanapplylocalderivativeoperatorstothescale-spacerepresentation:
DuetothecommutativepropertybetweenthederivativeoperatorandtheGaussiansmoothingoperator,suchscale-spacederivativescanequivalentlybecomputedbyconvolvingtheoriginalimagewithGaussianderivativeoperators.ForthisreasontheyareoftenalsoreferredtoasGaussianderivatives:
Interestingly,theuniquenessoftheGaussianderivativeoperatorsaslocaloperationsderivedfromascale-spacerepresentationcanbeobtainedbysimilaraxiomaticderivationsasareusedforderivingtheuniquenessoftheGaussiankernelforscale-spacesmoothing.[3][16]
TheseGaussianderivativeoperatorscaninturnbecombinedbylinearornon-linearoperatorsintoalargervarietyofdifferenttypesoffeaturedetectors,whichinmanycasescanbewellmodelledbydifferentialgeometry.Specifically,invariance(ormoreappropriatelycovariance)tolocalgeometrictransformations,suchasrotationsorlocalaffinetransformations,canbeobtainedbyconsideringdifferentialinvariantsundertheappropriateclassoftransformationsoralternativelybynormalizingtheGaussianderivativeoperatorstoalocallydeterminedcoordinateframedeterminedfrome.g.apreferredorientationintheimagedomainorbyapplyingapreferredlocalaffinetransformationtoalocalimagepatch(seethearticleonaffineshapeadaptationforfurtherdetails).
WhenGaussianderivativeoperatorsanddifferentialinvariantsareusedinthiswayasbasicfeaturedetectorsatmultiplescales,theuncommittedfirststagesofvisualprocessingareoftenreferredtoasavisualfront-end.Thisoverallframeworkhasbeenappliedtoalargevarietyofproblemsincomputervision,includingfeaturedetection,featureclassification,imagesegmentation,imagematching,motionestimation,computationof